Introduction
Customer retention remains a central challenge for modern businesses, especially in highly competitive markets. Companies increasingly turn to data-driven solutions to anticipate customer behavior and make strategic decisions that reduce revenue loss. One of the most powerful tools is churn prediction, which identifies customers at risk of leaving a service or product, which could make a great difference in your overall growth - according to a Bain & Company report, increasing customer retention rates by 5% can increase profits by 25% to 95%.
With the integration of artificial intelligence (AI), and particularly machine learning (ML), churn prediction has evolved from basic statistical models to sophisticated systems that can predict churn with remarkable accuracy. This article explores churn prediction, how AI enhances accuracy, which models are most effective, and why implementing AI-driven churn prediction can transform business decision-making.
What is churn prediction?
Churn prediction refers to the process of identifying customers who are likely to stop using a company’s product or service within a specific timeframe. This proactive strategy enables companies to intervene before customers exit, allowing them to deploy targeted retention efforts such as personalized offers, improved service delivery, or adjusted pricing strategies.
The concept of churn—also known as customer attrition—can be categorized into two types:
- Voluntary churn when a customer chooses to leave.
- Involuntary churn occurs when the customer is lost due to factors such as payment failures or subscription expirations.
Accurate churn prediction enables organizations to shift from reactive retention to proactive engagement, thereby reducing customer acquisition costs and maximizing lifetime value. According to Harvard Business Review, acquiring a new customer can be 5 to 25 times more expensive than retaining an existing one. Hence, effective churn management enhances profitability by reducing acquisition costs and increasing the customer lifetime value (CLV).
How does churn prediction work on AI?
Artificial intelligence, particularly machine learning, plays a central role in modern churn prediction systems. Rather than relying solely on historical averages or business rules, AI enables using complex models that analyze vast datasets to detect patterns that precede customer departure.
The data pipeline
An effective AI-powered churn prediction pipeline typically includes the following stages:
- Data collection: This includes transactional data, behavioral metrics, customer service interactions, demographic information, and engagement data.
- Feature engineering: Variables such as frequency of usage, recency of interactions, and changes in user behavior are extracted or constructed to serve as model inputs.
- Model training: Machine learning algorithms are trained on historical data where the outcome (churned vs. retained) is known.
- Prediction: The model estimates the probability of churn for each customer.
- Actionable insights: High-risk customers are flagged, allowing targeted retention strategies.
Importantly, many modern systems use supervised learning methods, where labeled data (i.e., historical churn outcomes) are available.

Main models and applications
Several machine learning algorithms are commonly applied in churn prediction, each with specific advantages depending on data structure, volume, and business requirements:
1. Logistic Regression
A foundational statistical model that remains widely used due to its interpretability, it predicts the probability of churn as a function of various customer features. Due to its simplicity and speed, it is frequently used as a baseline in churn prediction. One of the key advantages of logistic regression is its ability to produce probability estimates for customer churn, which are easily interpretable by business analysts and non-technical stakeholders.
2. Decision Trees and Random Forests
Tree-based models offer both interpretability and robustness to overfitting. They are particularly useful in churn prediction because they can capture non-linear relationships between features and the churn outcome while remaining visually interpretable. Decision trees handle both numerical and categorical variables without the need for extensive normalization or scaling. Trees provide clear rankings of feature importance, offering valuable insights into the primary drivers of churn.
Random forests combine multiple decision trees to improve accuracy and handle imbalanced data well. They combine the predictions of multiple decision trees built on bootstrapped subsets of the data with random feature selection at each split. This ensemble approach reduces overfitting, increases generalization capability, and results in higher accuracy and stability than a single decision tree. In the context of churn prediction, Random Forest offers robust performance across different customer segments and churn scenarios, including cases with imbalanced data.
3. Gradient Boosting Machines (GBM) and XGBoost
Among the most accurate and widely adopted models for churn prediction. XGBoost (Extreme Gradient Boosting) is particularly noted for its efficiency and predictive performance. It handles missing data gracefully and supports regularization, making it ideal for enterprise-scale churn prediction systems. They are widely used for churn prediction due to their exceptional predictive accuracy, scalability, and ability to handle structured data effectively.
4. Neural Networks
Deep learning models can automatically learn complex patterns and non-linear relationships in large datasets. However, they require more data and computational resources and are generally less interpretable than tree-based models.
Real-world applications
- Subscription-based platforms use AI churn prediction to tailor messaging and offers.
- Retail and e-commerce businesses personalize product recommendations and loyalty incentives.
- Telecommunications firms prioritize retention efforts based on churn probability.
- Financial services segment customers based on engagement patterns and transaction anomalies.
Take a look at Chargebee’s “Churn Watch” dashboard:
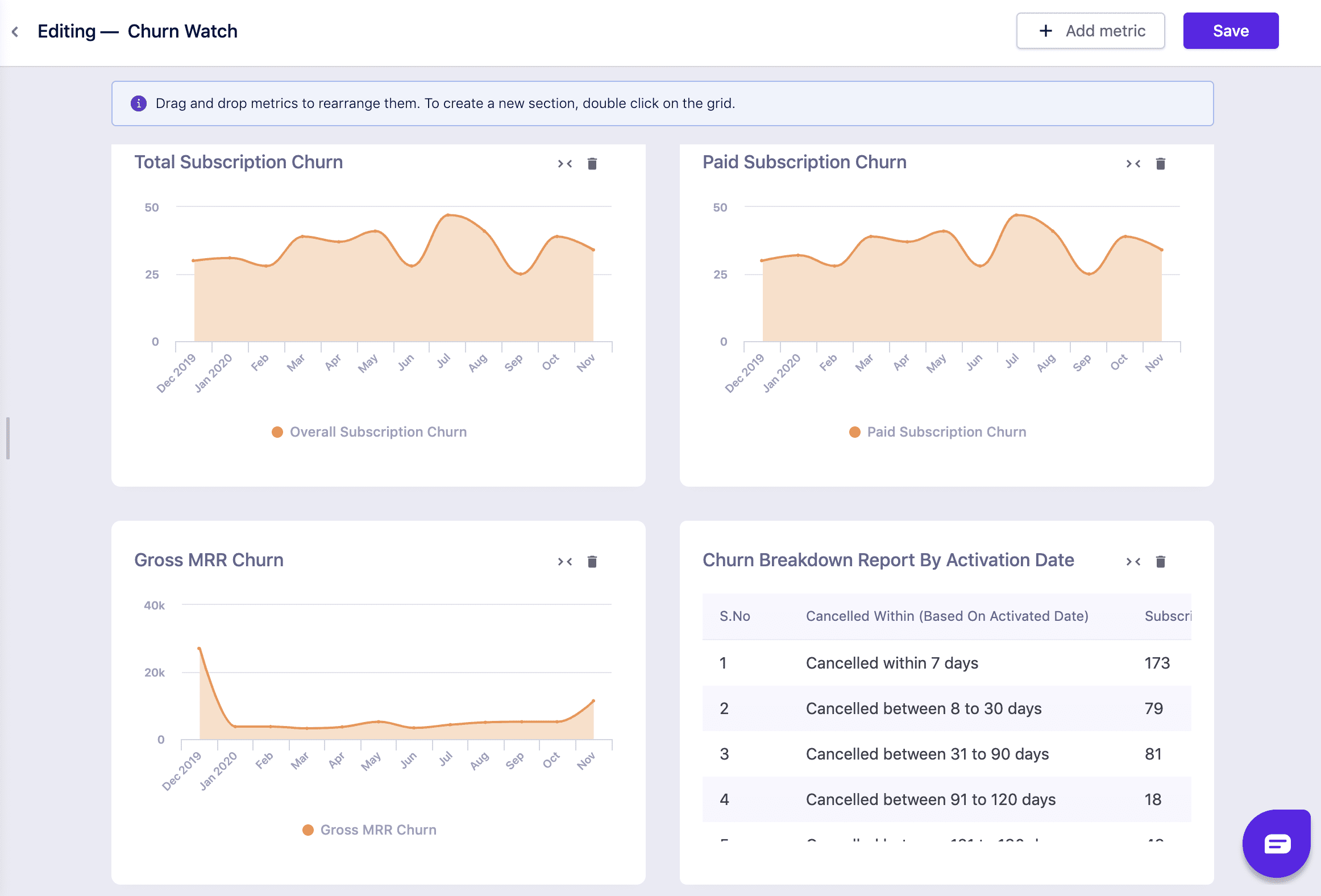
At Digital Sense, we have implemented churn prediction systems using advanced AI pipelines for companies such as Tonal, significantly improving customer retention rates. Through their dashboard, everything is set and ready for the team to interact with the data, filter the information and make decisions in their best makreting interests.
Benefits of implementing AI churn prediction
AI-driven churn prediction is not just a technical upgrade but a strategic business capability. Some of its key benefits include:
1. Improved customer retention
By identifying high-risk customers early, companies can take timely and personalized actions, reducing churn and increasing customer lifetime value.
2. Efficient resource allocation
Predictive models allow businesses to focus retention resources—like discounts or customer service follow-ups—on those most likely to churn rather than applying blanket strategies.
3. Better product development
By understanding churn drivers, businesses can improve their offerings. For instance, frequent app crashes or poor onboarding experiences can be addressed directly.
4. Enhanced marketing personalization
Estimated churn probabilities can be used as features in broader marketing automation systems, leading to more effective segmentation and targeting.
5. Quantifiable business impact
Companies that adopt AI-based churn prediction report up to 15–20% improvement in retention metrics within the first year of deployment.
6. Decision-making with probability
One of the most underappreciated benefits of churn prediction is the availability of churn probability scores. These scores are not binary decisions but continuous probabilities that can inform more nuanced business strategies:
- Adjusting service tiers for medium-risk customers
- Offering limited-time promotions for high-risk segments
- Deferring aggressive marketing for low-risk, high-value customers
Conclusion
When powered by artificial intelligence, churn prediction offers an advanced and data-driven approach to customer retention. With proven machine learning techniques like XGBoost, companies can make informed decisions that protect revenue, improve customer experience, and increase overall efficiency. To continue exploring AI for Marketing, explore what is Marketing Mix Modeling and demand forecasting, great ways to continue exploring you data efforts.
At Digital Sense, we specialize in building sophisticated AI solutions tailored to your organization’s unique needs. Whether you want to implement a churn prediction system or integrate it into a broader AI transformation strategy, our expert team is ready to help.
Explore our case studies, visit www.digitalsense.ai, or schedule a call with our team to learn how churn prediction can make a measurable difference for your business.