Traditional predictive models in marketing focus on estimating a customer’s likelihood of making a purchase. These models, often referred to as propensity models, help businesses identify high-probability buyers. However, a significant limitation of these models is that they do not account for the impact of marketing interventions. Many individuals targeted with these methods would have purchased regardless of receiving a promotion or incentive, leading to inefficient marketing spend.
Uplift modeling addresses this inefficiency by optimizing incremental sales rather than absolute shopping propensity. It identifies individuals whose behavior can be influenced by an intervention—whether a discount, advertisement, or loyalty reward. This approach ensures that marketing efforts focus on persuadable customers rather than those who would have converted anyway, maximizing return on investment (ROI) and improving campaign efficiency.
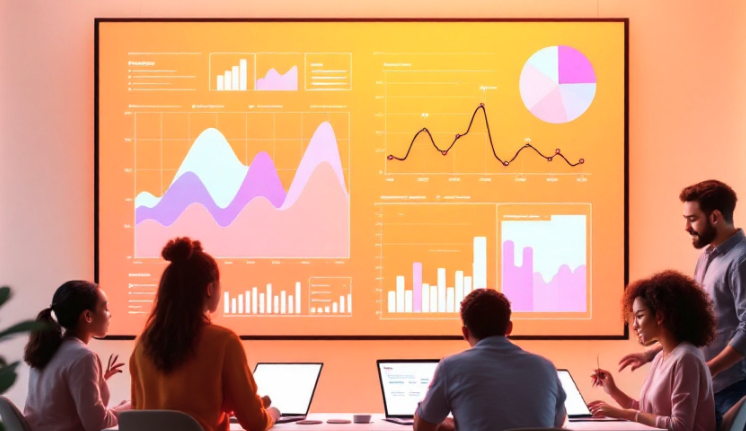
Previous Alternatives to Uplift Modeling
Before uplift modeling, marketers relied on standard propensity models or A/B testing alone to optimize campaigns. These methods had significant drawbacks:
- Propensity Models: While effective in identifying high-value customers, they do not distinguish between those who will purchase regardless of marketing efforts and those who need an additional push.
- A/B Testing: This traditional method measures the impact of a treatment versus a control group but does not provide a mechanism to predict who is most likely to be influenced by an intervention.
- Random Targeting: In cases where no predictive model was available, businesses often resorted to blanket promotions, leading to suboptimal resource allocation.
What is Uplift Modeling, and Key Differences with Previous Alternatives
Uplift modeling is a machine learning approach that estimates the causal effect of a marketing treatment on an individual basis. Instead of merely predicting whether a customer will buy, it predicts whether a customer is more likely to buy because of the marketing action.
A key component of uplift modeling is segmenting customers into four distinct groups based on their likelihood to convert with and without marketing intervention:
- Persuadables – Customers who will convert only if targeted. This is the ideal group for marketing campaigns.
- Sure Things – Customers who will convert regardless of marketing efforts. Sending promotions to them is inefficient.
- Lost Causes – Customers who won’t convert, even with marketing. Targeting them is a waste of resources.
- Sleeping Dogs – Customers who would buy only if NOT targeted. Marketing actions may have a negative effect on this group.

The key differences between uplift modeling and previous approaches include:
- Focus on Persuadables: Unlike propensity models that target likely buyers, uplift modeling identifies customers who will buy if and only if they receive the promotion.
- Causal Inference: Uplift modeling applies causal inference techniques rather than mere correlation-based predictions.
- Optimized Marketing Spend: Uplift modeling significantly reduces waste in marketing budgets while increasing incremental sales by targeting only persuadable customers.
Most Common Approaches for Uplift Modeling
Several machine learning techniques have been developed to estimate uplift, each with strengths and limitations. Among the most widely used methods are Uplift Trees and Uplift Random Forests, which extend traditional decision trees to optimize treatment effect estimation. Meta-Learners—including the T-Learner (Two-Model Approach), S-Learner, and X-Learner—offer different strategies for modeling individual treatment effects based on standard predictive models. While each approach has its merits, as an example, in this article, we will focus on the T-Learner / Two-Model Approach.
T-Learner
One of the most commonly used methods for uplift modeling is the T-Learner, a Two-Models approach. This technique involves training two separate machine learning models:
- Treatment Model: Trained on data from customers who received the marketing intervention.
- Control Model: Trained on data from customers who did not receive the intervention.
The uplift score for each customer is then calculated as the difference between the treatment model’s and the control model’s predictions. This allows businesses to identify individuals who positively respond to the marketing action.
Success Story: Uplift Modeling for retail
At Digital Sense, we implemented an uplift modeling solution for a large US-based retailer. The objective was to improve marketing efficiency by identifying customers who would purchase only if they received a promotional offer.
Methodology
We designed a model that classifies customers into three segments based on engagement levels:
- Highly Engaged: Likely to purchase regardless of promotions.
- Persuadables: Customers who will only purchase if they receive a promotion.
- Non-Responsive: Unlikely to purchase even with an incentive.
Our approach included:
- Data Cleaning & Feature Selection: Starting with the top features from existing propensity models, we iteratively refined feature importance using Causal Gain (CGain) analysis on a validation set.
- Model Training: We implemented a T-Learner approach using the CausalML library. The classifiers used by the learner were Random Forest Classifiers, providing a balance between model complexity and generalization.
- A/B Testing: We validated the model in a real-world scenario by splitting customers into a control group (no treatment) and a treatment group (promotion received). The goal was to measure the true impact of targeted interventions.
Who did we target?
After evaluating the results of our Uplift model, we observed a significant shift in the customer segments selected for targeting compared to the previous propensity-based approach. In the prior model, the majority of targeted customers belonged to the highest engagement tier. However, our uplift model identified this group as the smallest proportion among the selected customers. Instead, the model prioritized targeting customers from the lowest engagement tier. This outcome aligns with our expectations, as high-engagement customers are likely to make a purchase regardless of marketing efforts. By focusing on persuadable customers, our model effectively maximized incremental sales. We validated this approach in practice by deploying the model in a subsequent campaign and measuring its impact through an A/B testing strategy.
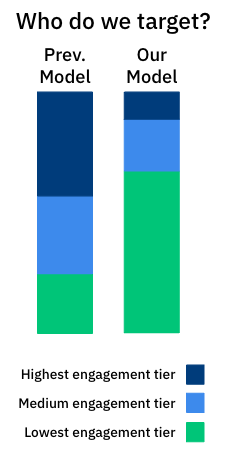
Results
Our uplift model significantly outperformed previous methods:
- +150% Incremental Margin compared to previous targeting methods.
- +50% Marketing ROI, demonstrating significantly improved efficiency in promotional spending.
- +30% Incremental Sales, meaning a much higher proportion of sales were directly attributable to the targeted marketing efforts.
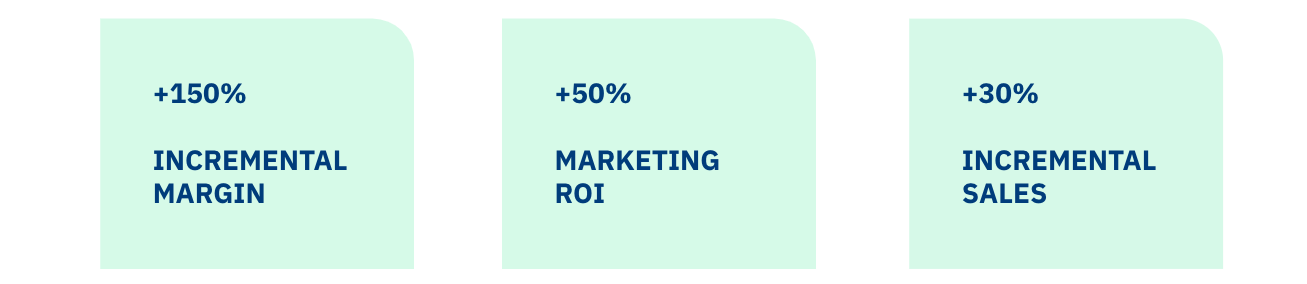
Tools and Best Practices
When implementing uplift modeling, businesses should consider the following best practices:
- Use Causal Inference Techniques: CausalML, DoWhy, and EconML can help estimate causal effects more accurately.
- Leverage Machine Learning Algorithms: Tree-based models such as Random Forest and XGBoost are highly effective in learning non-linear relationships.
- Iterative Feature Selection: Feature importance should be continuously refined using validation sets to maximize predictive power.
- Ensure Proper A/B Testing Design: Always include control and treatment groups to validate uplift model effectiveness before deployment.
- Optimize for Persuadables: Avoid wasting marketing spend on customers who would purchase regardless of intervention.
Conclusion
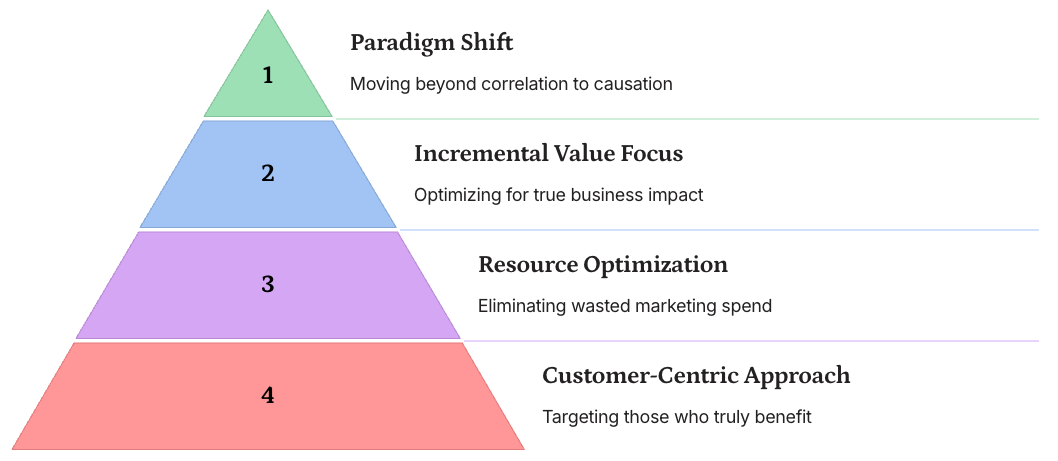
Uplift modeling represents a paradigm shift in targeted marketing. It allows businesses to move beyond simple propensity models and focus on incremental sales optimization. Companies can significantly enhance marketing efficiency and ROI by leveraging causal inference and machine learning.
At Digital Sense, we specialize in developing advanced AI-driven solutions tailored to business needs. Our expertise in machine learning, causal inference, and data science ensures our clients stay ahead in an increasingly competitive market. If you are still exploring data science, we encourage you to check out our article: what is data science?
If you want to optimize your marketing strategy with cutting-edge AI techniques, visit our website and discover more about our data science consulting services.