In today’s complex marketing landscape, companies invest in multiple channels—TV, social media, digital ads, email campaigns, and more. But how can they determine which investments are driving sales? That’s where Marketing Mix Modeling (MMM) comes in.
MMM helps businesses analyze their past marketing efforts to see what works best, ensuring smarter decisions and better returns on investment. Using advanced machine learning techniques, MMM forecasts the impact of marketing decisions and optimizes the marketing strategy to achieve sales, revenue, profit, or other business goals. This guide will explain MMM step by step, provide real-world examples, and compare it with other marketing attribution models like Multi-Touch Attribution (MTA).
What is Marketing Mix Modeling (MMM)?
Marketing Mix Modeling uses statistical analysis and machine learning techniques to help businesses measure the impact of different marketing activities on sales or other key performance indicators. It considers various factors—advertising, promotions, seasonality, pricing, and even economic conditions—to determine how much each contributes to business performance. It can also be used to forecast the performance of marketing initiatives, simulate future performance, and optimize marketing campaigns.
What Does MMM Do?
MMM helps businesses:
- Identify the most effective marketing channels (TV, online ads, in-store promotions, etc.).
- Optimize marketing budgets by redistributing spending to the highest-performing channels.
- Forecast future sales based on different marketing strategies.
- Adjust strategies based on external factors like inflation, competitor actions, or new product launches.
How Does MMM Work? A Step-by-Step Guide
Step 1: Collect Data
When it comes to machine learning, data is truly important. To truly understand what is machine learning and its importance in Marketing Mix Modeling, a good look into data is required. MMM requires historical data from multiple sources:
- Marketing data: Ad spend on different platforms (TV, digital, print, social media, radio, etc.).
- Sales data: Revenue, units sold, and profit margins.
- External factors: Competitor pricing, economic trends, seasonal effects (e.g., holiday shopping spikes).
- Non-marketing influences: Product launches, customer reviews, or distribution changes.
Example: A beverage company wants to understand how much each of its marketing efforts contributes to overall sales. It collects data on TV commercials, social media ads, billboards, and in-store promotions over the past two years.
Step 2: Analyze the Data
Once data is collected, statistical models (often regression analysis) are used to identify patterns and estimate the contribution of each marketing activity.
Example: After analysis, the beverage company finds that:
- TV ads drive long-term brand awareness but have a delayed effect on sales.
- In-store promotions boost short-term sales but don’t create lasting brand loyalty.
- Digital ads drive immediate and long-term sales, making them a high-return channel.
Step 3: Build the Model
A mathematical equation is created to represent how marketing efforts impact sales. It looks something like this:

Each variable gets a weight based on its impact.
Example: If the beverage company’s model shows that social media ads have the highest impact (0.5), they may increase spending there while reducing investment in TV ads.
Step 4: Generate Insights and Adjust Marketing Strategy
Based on the results, businesses can:
- Shift budgets to the most effective marketing channels.
- Test different strategies (e.g., reducing TV ads and increasing digital spend).
- Forecast future performance under different scenarios, for example, using machine learning techniques.
Example: The beverage company decides to:
- Reduce TV ad spending by 20%.
- Increase Instagram and TikTok ads by 30%.
- Launch more in-store promotions during peak summer months.
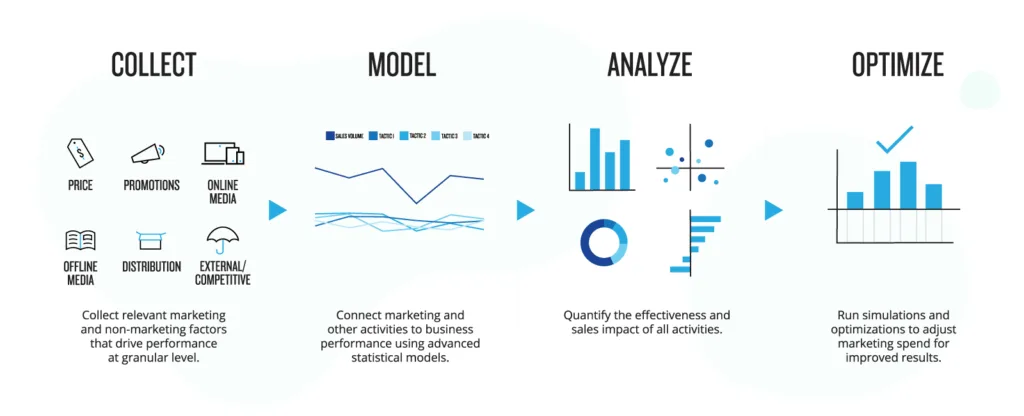
Why is MMM Useful?
Marketing Mix Modeling is essential for data-driven decision-making. Its benefits include:
- Budget Optimization: Ensures money is spent on high-impact channels.
- Long-Term Strategy Planning: Helps businesses move beyond short-term performance metrics.
- Holistic View: Considers both digital and offline marketing channels.
- Predictive Insights: Enables forecasting and scenario planning.
Example: A car manufacturer used MMM and discovered that radio ads had a stronger influence on older consumers than digital ads. As a result, they adjusted their media strategy by increasing radio spending in key regions.
MMM vs. Multi-Touch Attribution (MTA)
While both MMM and MTA help measure marketing impact, they have different approaches.
Example:
- MMM Use Case: A supermarket chain wants to know how TV ads, flyer promotions, and social media campaigns drive total revenue.
- MTA Use Case: A clothing brand wants to track which digital ads (Google, Facebook, Instagram) lead to the most online purchases.
When to Use MMM vs. MTA?
- Use MMM when analyzing both online and offline marketing efforts.
- Use MTA for digital-only campaigns where user-level tracking is possible.
- Combine Both for a complete view of marketing effectiveness.
Conclusion
MMM is a powerful tool for businesses looking to optimize their marketing strategies. By analyzing historical data, MMM helps companies understand what works and what doesn’t and how to allocate their budgets more effectively.
While Multi-Touch Attribution focuses on digital ads, MMM provides a broader, long-term perspective that includes traditional marketing channels. Companies that leverage MMM can make better, data-driven decisions, improving their marketing ROI and staying ahead of the competition. The possibilities with AI, taking machine learning and data science to its limits, can potentiate any business decision. Take for example our churn predicition case with tonal, plus the ultimate guideline to customer churn prediction.
If you are looking to optimize your marketing efforts, contact us and we can discuss how we can use AI to elevate your marketin ROI and growth efforts.